2016 USWNT – Blame it on the Rio Olympics- Should Have This Team Been There? Part 2- “KISS Ratings”
By Steve Fowler
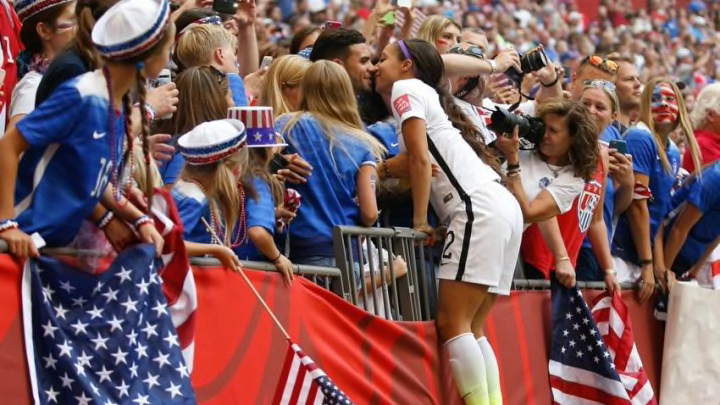
In a previous review of the USWNT players at the 2016 Rio Olympics, I looked at all the NWSL players’ statistics using a model proposed by McHale, Scarf, and Folker. This was an effort to determine whether the best players statistically were at the Olympics or not.
In this review, I will be looking at players using “KISS”.
No, I am not talking about Paul Stanley, Gene Simmons, Ace Frehley, and Peter Criss. I am not talking about what French people supposedly do. I am talking about “Keep It Simple Stupid!”. However, “KISS” is not the “official name” for this calculation. But this was the best informal name that I could come up with.
(Do you really want to read about something called “Fowler’s Simplistic Ranking Statistical Formulation for Soccer Player Ratings”, or FSRSFSPR, for short? Me neither! That’s why I called it “KISS”!)
Stat Geek Warning! This article contains over 3000 words. So, if you do not like statistics, do not waste your valuable time here, unless you want to try the link below to the animated series on the U.S. National Team players listed. And there are other bonus videos at the bottom.
(Here is a set of videos made for the USWNT players (and coach Jill Ellis) just before the Olympics. Some of them are quite funny. This will help break up the monotony of statistical analysis. Hopefully, this link works.)
http://www.ussoccer.com/womens-national-team/2016-wnt-animated
The McHale, Scarf, and Folker (2012) statistical model had a few flaws as goalkeepers seemed to be random. And in the math, fullbacks were more valuable than center backs. I attempted to modify their calculations with some success. From there, I tried to calculate who should or should not be on the U.S. National Team. But because I cannot verify my calculations statistically, my results may be better or worse than using McHale et al.. This is a link to that review.
In this analysis, however, I will be taking a simpler approach to the statistics. I will be ranking the general categories of passing skills, defensive skills, set piece skills, possession skills, and overall playing time.
In general, I will be using the same mid-year 2016 statistics that Alfredo Martinez Jr. has at WoSo Stats, and taking NWSL league data as well. I will be making several categories, and ranking the players within the categories. Except, it will be an “upside down rank”, where first place will get 205 points (the approximate number of players studied).
I will be adding up those ranks and making a grand total. I will divide by the number of parameters studied to make an overall category score. Then I will then add all the categories together to make a sum to determine the “ultimate players” in the first half of 2016 after ranking all the players together.
The “KISS” Calculation.
Simply add each of these 5 category scores together.
1. Defensive skills
8 parameters were ranked for defensive skills of a player. In most cases, the higher the number increases your ranking points.
- Blocks per 90 minutes.
- Clearances per 90 minutes. (Getting the ball out of trouble near your goal.)
- Interceptions per 90 minutes.
- Opp. (Opposing) Balls Disrupted per 90 minutes.
- Opp. Possession Disrupted per 90 minutes.
- Tackles (Total).
- Opp. Passing Completion percent. This is an “upside down” scoring rank. Obviously you want your opponent to have a low completion rate, therefore the higher your score.
- Dribbled per 90 minutes. “Dribbled” means how many times an opponent gets past you. Also, for this parameter, a lower score will result in higher points.
Again, for these calculations, players were scored from 1 to 205. “205” means that you are the best in that particular category. All of the scores were added up, and divided by 8 to give a “Defensive skills” score.
2. Playing Time
It is one simple calculation. Minutes played divided by “total team minutes”. All players are then ranked according to how much time they are on the pitch. This is to reward all players with significant playing time to help offset the “bench players” who might have high percentages in certain parameters, but limited playing time.
3. Passing Skills.
6 parameters studied here.
- Cross completion percent.
- Key passes per 90 minutes. (A pass that leads to a shot at goal.)
- Launch (or long ball) pass completion percent.
- Through ball completion percent.
- Passing attempts per 90 minutes.
- Passing completion percent.
All are pretty much self-explanatory and straightforward. Like the other categories, add them up and divide by number of parameters.
4. Set piece skills
- Throw In attempts per 90 minutes.
- Throw in completion percent.
- Free kicks attempted
- Free kick completion percent.
- Corner Kicks Taken
- Corner Kick completion percent.
The ‘attempts’ were to offset the ‘percentages’. So, in a sense, a person “persevering” was rewarded over a player only having one or two attempts, but having a higher completion percent.
5. Dribbling / Possession skills
4 parameters to see how well you maintain possession.
- Aerial Duels win percent. (Basically, out-competing your opponent for “headers”, etc.)
- Take-on win percent. (Dribbling around your opponent.)
- Take-ons won per 90 minutes.
- Possessions disrupted per 90 minutes. This is similar to losing a “take on”, but has other factors in its calculation, such as lost touches, or any other dispossession. This is an “upside down score” as well. The lower your number means that you will receive more ranking points.
Failed efforts in “KISS”
I tried to incorporate goalkeeping data for all the players. This was to hopefully establish goalkeepers on the same level with other players, and compare passing skills, etc. I looked at things such as ranking goals against average and save percentage. It still made the goalkeepers “random”, there was not enough data in other categories to bring up goalkeepers.
I also looked at offensive statistics such as goals scored, assists, and shot percentage. Anyone not scoring a goal or assist was severely “punished”. Therefore, any player scoring a goal had inflated rankings. So, the National team, according to this method, would have been comprised of only those who scored goals or assists this past NWSL season..
Therefore, the above 2 categories of goalkeeping and scoring were eliminated. So, we added up the 5 other categories above and …
Drum roll, please….. As a reminder, here were the players invited to the Rio Olympics in 2016. The number on the left is their total score when all 5 categories were added up. The number on the right is their overall rank of all NWSL players in the first half of 2016.
Goalkeepers
- Hope Solo 454 / 108
- Alyssa Naeher. 402 / 128
Defenders
- Whitney Engen 657 / 23
- Julie Johnston 612 / 43
- Megan Klingenberg 620 / 41
- Ali Krieger 698 / 11
- Becky Sauerbrunn 753 / 2
- Kelly O’Hara 706 / 9
Midfielders
- Morgan Brian 673 / 19
- Tobin Heath 632 / 35
- Lindsey Horan 641 / 32
- Carli Lloyd 257 / 177
- Allie Long 731 / 5
- Megan Rapinoe 0 / 0
Forwards
- Alex Morgan 524 / 79
- Crystal Dunn 592 / 49
- Christen Press 522 / 82
- Mallory Pugh 0 / 0
Alternates
- Heather O’Reilly 597 / 47
- Emily Sonnett 570 / 63
- Ashlyn Harris 433 / 115
- Sam Mewis 635 / 30
Again, the goalkeepers have “random data”, and this method does not rank them in a viable way, so my conclusions would be the same as on my previous post. Also, my assessments from the previous post on Lloyd, Pugh, and Rapinoe are the same since their overall data was not changed.
These players have good overall statistics and should be on the USWNT.
- Becky Sauerbrunn. According to my math, she is the number one player in the defensive skills department. She also does quite well in the passing skills, and dribbling skills areas also. With the McHale et al. calculation it emphasized the “attacking player”, which Sauerbrunn clearly is not at the center back position, and she was previously ranked 58.. Despite some uncharacteristic miscues early in the season, she returns to the top of the defensive player pantheon with this calculation.
- Allie Long. She is now top 5 with this calculation. And this calculation has nothing to do with overall team statistics. So, here Long’s high-ranking has nothing to do with Portland, as with the case in the original McHale et al. calculation. She is average defensively but almost top 20 in the other categories. (So, why is she a center back in the 3-back version of the National Team defense now?)
- Ali Krieger. Just as I guessed! In my previous analysis as I had no data to support it, but I estimated Krieger could be a top 15 player. It is well-known fact that Krieger has been one of the best defenders on the U.S. National team for nearly a decade. She is ranked 5th overall in defense, and near top 25 in the other categories. So, why did she sit on the bench during the quarterfinal loss?
- Morgan Brian. She is now a top 20 player overall using the “KISS” calculation. She is ranked 12th defensively, and has superior possession skills. In this estimate, like the McHale et al. calculation, she is hurt mathematically by missing some time to injury.
(Misjudged once again statistics don’t lie.)
- Kelly O’Hara. I partially apologized to her in my previous review, and now I must apologize again. She is ranked number 9 overall, helped by her defensive skills at ranking 4. Her attacking skills as calculated using McHale et al. cement her in the top 10. And in the “KISS” calculation, her defensive abilities are good enough to put her on the National Team as well.
The “KISS Rating” Hurts Them a Little, But They Are Still “Okay”
- Tobin Heath falls to 35 in this overall rating. Ironically, she was asked to play defense in the quarterfinal loss to Sweden, and here she is quite low for a starting player defensively at rank 80. In a previous article, I complained that she tried to dribble around too many defenders unsuccessfully, and that shows up here as she is ranked 106 for possession skills . She is quite strong at rank 11 for set pieces. Which is funny in a way, as Lloyd took the ball from her during the quarterfinal and promptly missed wide left of goal. Maybe next time, Coach Ellis and Captain Lloyd will let the players play to their strengths?
- Sam Mewis falls to 30 from 17 in my previous analysis. With McHale et al. calculation she is buoyed by the “attacking pass” calculation as well as well as 2 goals and 2 assists that were part of the original statistics. Here, her passing skills are top 20, but she is average at other categories, but is helped by playing time as well.
- Crystal Dunn – the “KISS calculation” to me seems worse for forwards overall compared to the “modified McHale et al. calculation”, where I added “attacking passes” as opposed to “crosses only” to help forwards in that calculation. Here, Dunn is the second best forward (the better player is listed below). Dunn falls to rank 49, which is far better than her comrades Press and Morgan.
These Players are Still “Maybe’s”
- Whitney Engen. She is ranked 23 overall. However, Engen is an average defender at rank 46, outranked defensively by teammates King (defensive ranking at number 3!), Kallman, Elby, and Pathman on Boston. She makes the grade due to her superior passing and dribbling skills which are near top 15.
- Lindsey Horan. She is ranked 32 here and there are better midfielders about. The problem with Horan in this calculation is that she is quite good in passing ranked 10, but is 33rd in set pieces, and not even ranked top 50 elsewhere. But in the quarterfinal against Sweden, not many passes penetrated their bunker defense.
- Alex Morgan. The math again does not work for Morgan as she is ranked 79. But again, forwards have no “statistical love” in this calculation.
- Christen Press. She is slightly behind Morgan. With the lack of “good” statistics for Press and Morgan, it is difficult to see them dominating on the National Team for the next few years. Both Press and Morgan need to work on their passing and possession skills to stay on top of the National Team hierarchy, as younger more skilled players could soon pass them by. And that neither are good defensively hurts them as well.
- Julie Johnston– Johnston probably stays on the team in this rating as she is the 19th ranked defender,and is top 15 in possession skills. But her passing skills are not the best at rank 99.
Maybe They Should Have Stayed Home?
- Heather O’Reilly. She is ranked 47, but has no top 50 skills other than set pieces.
- Emily Sonnett. Here her rank is nearly the same as the ones performed using the McHale et al. systems. She is an average player ranked at 63. Unfortunately for her, there are lots of defenders ranked ahead of her.
- Megan Klingenberg. She is ranked 41, and like Sonnett, there are others in her position that rank ahead of her. She is also ranked 54th defensively, which is not very good considering there are only ten teams in the NWSL. So, she is being to compared to 39 other starting defenders, plus about a dozen starting defensive midfielders.
These Players Should Have Been Considered (Again)
- Vanessa DiBernardo. The Chicago midfielder again makes this list ranked 4th overall at 734 points. Her strongest categories are possession, defense and set pieces, all in the top 17.
- Lauren Barnes. She is again near the top of the overall list, this time at the top at number 1. Ironically, the NWSL Defender of the Year is average defensively, ranked 57th. But all of her other skills are near top 10.
- Sarah Killion. The Sky Blue midfielder is here again ranked in the top 10 at number 8. She is ranked top 15 in both possession and passing.
- Liz Eddy. Again, she is among the best at fullbacks, ranked ahead of new U.S. National team favorite Casey Short. However, her numbers are not spectacular, but is ranked 18th overall defensively, and very average in passing and dribbling. Her high-ranking is due to the set piece plays (number 5 in that category). But even so, she is still ranked better than players like Klingenberg and Sonnett.
- Casey Short and Arin Gilliland. While Short (rank 31) has been receiving playing time on the National Team, her teammate Gilliland is just ahead of her in the ratings once again (ranked 28). While Gilliland is a top 6 defender, Short is the better all-purpose player, ranked top 40 in almost all categories, except set pieces.
New Faces To Consider?
- Dani Colaprico. She gets mentioned here, being ranked 3rd at 739 points, just ahead of her teammate DiBernardo. She was ranked about 35 using the McHale et al. system Here, she is the second best defender in this system and 6th best passer. She was recently called to the National Team but has not received many opportunities to play. Unfortunately, with Coach Ellis seemingly abandoning the idea for anything resembling defense, I have my doubts that fine defensive midfielders like Colaprico will play much for the National Team.
- Beverly Yanez. The Seattle forward/midfielder gets consideration using the “KISS calculation”. She is ranked 17 overall at 681 points, but she is an average dribbler and defender, although her passing skills are top 10. And she is pretty good at set pieces as well. Players like Lynn Williams fell to 89 using this calculation. So, maybe Yanez is severely underrated? Both players deserve consideration over Rapinoe and Lloyd who were disastrous during the quarterfinal loss.
- Brittany Taylor and Yael Averbuch, Both of Sauerbrunn’s teammates make the list thanks to both being top 20 players. Averbuch is good at possession at 18. Taylor is better defensively at 11. They are ranked 18 and 15 overall, but none of their other categories are outstanding.
Born in The Wrong Country Once Again
- Kim Little is ranked 6 on this list, slightly hurt statistically that she does not get credit for goals and assists here. She is the league’s best passer, and quite good at set pieces. However, her defense is ranked at 124 showing that she is a mortal.
Conclusions
The “KISS calculation” only took a few hours to execute total time, compared to several days worth working on McHale, Scarf, and Folker. There seem to be advantages and disadvantages to each system. McHale et al. favors the attacking player (regardless if I modify crosses into “attacking passes” or not.), where the “KISS system favors the defensive players. Neither system works at all with goalkeepers, and true forwards on both systems are rated low as well.
So, maybe having the 5 equal “KISS” categories might be the better way to determine the best all-purpose player. This is better than the original McHale, Scarf, and Folker system where crosses and team points were the most important factors. Modifying that calculation by having team points eliminated and with crosses changed to become “attacking passes”, the McHale et al. statistic improved as it included other players more equally. But the McHale et al. system seems flawed as certain “weighing coefficients or constants” (a.k.a “fudge factors”) appeared to be unnecessary, and did not affect overall rating numbers much when removed.
But, if you think about it, soccer is a low scoring sport. Therefore, defense should be rated highly to match what is being played on the field. So, in my opinion, the “KISS” calculation might be fairly accurate overview of a player’s net worth on the pitch.
Maybe another sequel?
However, I will be researching to see if there other alternative statistical means that will readily evaluate all the NWSL players using the statistics that I have available through WoSoStats and the NWSL League site. Perhaps, there is a statistical method that everyone can conclusively agree on the overall quality of a player. If not, I may try another “homemade” calculation, and see what the results are. Just for fun, I might name my next calculation – “SNAFU” – “Statistically Normalized – All For ‘U’!- but I am sure it will be a real SNAFU! 🙂
Here are a couple more videos.
Here is a great article by Stephanie Yang at The Bent Musket that includes an 8 minute video that documents the everyday life of Rachel Wood over the course of last winter. Wood was a substitute player on the Boston Breakers last season. But, she is a typical player that shows that for the lower paid players in the NWSL, it is a struggle financially for them to be able to play in the NWSL in order to pursue their dreams. Statistically, she was ranked 156 due to limited playing time. But she was the best player on the “KISS” list for possession skills.
http://www.thebentmusket.com/2016/8/22/12553990/rachel-wood-boston-breakers-nwsl-offseason
http://www.nbcolympics.com/news/us-women-fall-sweden-pks-eliminated-olympics
Sources:
- McHale, Scarf, and Folker: Soccer Player Performance Rating System , 2012 “On the Development of a Soccer Player Performance Rating System for the English Premier League” Interfaces Vol. 42, No. 4, July–August 2012, pp. 339–351 ISSN 0092-2102 (print)—ISSN 1526-551X (online). ©2012 INFORMS
- I have acquired about a half season’s worth of 2016 NWSL data from Alfredo Martinez, who has https://wosostats.wordpress.com and I also took the data from his report here.
- Some data is also taken from the NWSL site
- “KISS” https://en.wikipedia.org/wiki/KISS_principle.
- Here is a quick glossary for some of the terms above: https://www.whoscored.com/Glossary