2016 U.S. Women’s Soccer- Blame it on the Rio Olympics- Should Have This Team Been There?
By Steve Fowler
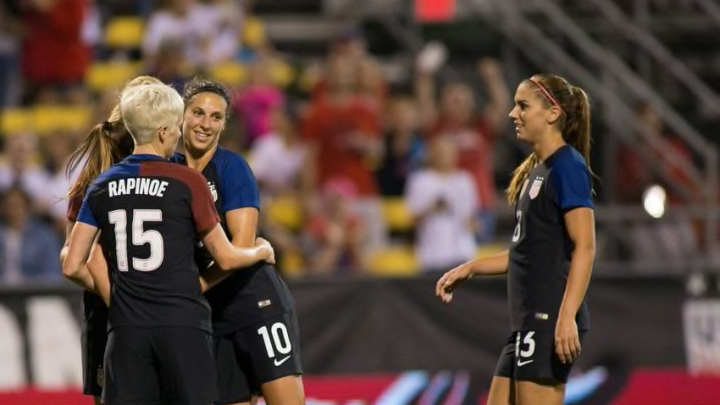
Should have Coach Jill Ellis picked the team that went to the Rio Olympics? Or should she have looked at alternative players? Here are some statistical analyses to determine whether the roster should have been changed.
Warning! This article contains over 3800 words describing statistics. Therefore, this article will be boring for well, everyone, except hardcore soccer nerds. This might take more than 15 minutes to read. Recommended for insomniacs.
This game went down as a disaster for the U.S. Women Soccer Team. They lost a quarterfinal match to Sweden in the 2016 Rio Olympics. There are dozens of commentaries that perfectly explained what happened with bizarre coaching decisions, players to blame, and the aftermath where Hope Solo was suspended 6 months for her famous “cowards” comment. I will not be rehashing these inglorious moments. Instead, I will be looking at some statistics to see if the 18 players and 4 alternates that were chosen deserved to be at the games in the first place. And who were possible candidates that could have been there, and who might have secured a victory.
Who was there? And why are there numbers by their name?
Goalkeepers
- Hope Solo 466 / 28
- Alyssa Naeher. 531 / 22
Defenders
- Whitney Engen 478 / 27
- Julie Johnston 450 / 31
- Megan Klingenberg 430 / 36
- Ali Krieger 427 / 38
- Becky Sauerbrunn 351 / 58
- Kelly O’Hara 681 / 7
Midfielders
- Morgan Brian 278 / 75
- Tobin Heath 866 / 2
- Lindsey Horan 445 / 32
- Carli Lloyd 41 / 159
- Allie Long 460 / 29
- Megan Rapinoe 0 / 0
Forwards
- Alex Morgan 276 / 76
- Crystal Dunn 597 / 16
- Christen Press 378 / 51
- Mallory Pugh 0 / 0
Alternates
- Heather O’Reilly 402 / 44
- Emily Sonnett 339 / 61
- Ashlyn Harris 650 / 12
- Sam Mewis 596 / 17
Now onto those mysterious numbers by their name…
The number on the left is an index total described below, and the number on the right is the overall NWSL ranking of the same index score out of about 205 players.
Before we begin, all statistics were taken from the NWSL website and from Alfredo Martinez Jr.’s data at Wosostats.wordpress.com and Tableau.Public.com. The calculations are mine, but is my interpretation of the McHale, Scarf, and Folker, 2012 paper in Interfaces Vol. 42, No. 4, July–August 2012, pp. 339–351 ISSN 0092-2102 (print)—ISSN 1526-551X (online) titled “On the Development of a Soccer Player Performance Rating System for the English Premier League”.
The calculation was based on McHale, Scarf, and Folker researching hundreds of English Premier League games and making statistical calculations to determine a player’s value for EA Sports®. I tried to match the statistics found in their paper with Mr. Martinez’s data. Some did not quite match perfectly, so I tried substituting a similar data for the sake of comparison. McHale et al. basically came up with a regression correlation that can be used to calculate a value based on 6 indices, such as goals and assists, minutes played compared to total time the team played, and a points sharing system where players on a winning team reap the benefits of victory mathematically, while losing teams do not receive as many points. Keep in mind that I am not a professional statistician, and everything below is my interpretation. The calculation is described more fully below:
More from U.S. Soccer
- Late Surge by USMNT in Nations League: Overcoming Challenges in Style
- Emma Hayes: The Right Choice to Lead the Triumphant Future of the USWNT
- USWNT Dominates Colombia in Convincing 3-0 Victory
- Tactical Analysis: USWNT Showcasing Defensive Dominance and Promising Offensive Potential Against Colombia
- CBF Proposes to Host the 2024 Libertadores Final in the USA
Index 1 “Player Contributions”
The first index is the most mathematically complex as it looks at things like crosses, dribbles, how many yellow and red cards the other team received, plus passes, and so on. A couple of items did not match with the data I had , so I substituted shots that were taken care of by the defender and goalie in lieu of cleared balls that McHale et al. used. There was a possession type ratio, so I calculated my ratio based on how times a player disrupted another player as compared to the times disposed by another.
Based on the regression data, the formula for Index 1 would be this:
Index 1 = (Crosses X 0.519) + (Dribbles X 0.118) + (Opp. Interceptions X (-0.024)) + (Opp. Yellow Cards X 0.253) + (Opp Red Cards X 0.1023) + (Opp Tackle Win Ratio X (-0.170) + (Opp Cleared X (-0.017))+ ( Passes X 0.034) + Constant of 6.463.
Rough translation of above: Crosses are very good. Dribbles are good, Having the ball taken away by the opponent (“Opp.” above) is bad either by interception or tackle. But getting your opponents in foul trouble with Yellow Cards and Red Cards is good. And of course passes are good. And anywhere you see the word “constant”, think “fudge factor”. An ideal regression should go through the Y axis at zero. But seldom in life are “ideal regressions”.
The numbers that are being multiplied above are “weights”, so crosses have much more value than ordinary passes. And with a minus sign, it is possible to have a negative index score.
Index 2 to Index 6.
There are 5 other indices where each may be multiplied by a factor.
Index 2 is the Points Sharing Index. What McHale et al. are doing here is rewarding victory or tie points to a player’s worth. This is 3 points per victory or 1 point per draw, times the minutes the player played in a game compared to the 90 minutes of a game. So, if your teams wins and you play the full game, you get full credit. Sit on the bench, you get nothing.
Index 3 is the Appearance Index. Index 3 is almost the same as Index 2, you simply get credit for playing whether you win or lose, and that is it. And you multiply by a “fudge factor” of 1.34. Note that this is for all games. However, I did not “punish” any players that were on U.S. National team duty for those games they missed for “friendlies”.
Index 4 is the amount of Goals Scored, times a factor of 1.039. Again, think “fudge factor”. But the totals of all players should be the same as the total number of goals scored in the league including weighing factors.
Index 5 gives credit to Assists. This is multiplied by the same factor of 1.039. The number of assists for the league should match the players total after calculations
Finally, the sixth Index is the Clean Sheet category. A clean sheet is where a team shuts out the opponent for the entire game. McHale et al. came up with 2.784 as a weighing factor, meaning almost the 3 points for a victory, but not quite since you can have a 0-0 draw. Then, each position gets a share. The goalie gets 58.5% of the share. The defender gets 36.4% share. The midfielders get 15%, and the forwards only get 7.1%. Again, the number of clean-sheets should match all of the points of all players in that category, except here you divide by 11 as all 11 players get credit for a clean sheet.
McHale et al. Final Calculation
Each of the 6 indices were added together with various “weights” to determine a total index value, which was multiplied by 100 for the sake of giving an approximate scale of 0 to about 1000.. Any player at 500 or higher is considered among the best.
Final Index= 100 X ((Index1 X 0.25) + (Index2 X 0.375) + (Index3 X 0.125) + (Index4 X 0.125) + (Index5 X 0.0625) + (Index6 X 0.0625).
After many hours of calculations, I then ranked the players based on this value to see who the NWSL’s top players for the first half of 2016.
Drum roll please….
And your U.S. National Team should be ……
The Portland Thorns!!!!!!!!!
Just kidding. 🙂
Part of the problem was that Mr. Martinez’s data is not “even”. Most teams had played about 10 games, where Portland had “played” 14 games. Therefore, more “weight” was given to the Portland players as they had won more games. But also, the players would have more chances to score more goals and assists.. But since several U.S. National players come from Portland, it was difficult to see if they “deserved” the justification of going to the Olympics.
When I looked at goalkeepers data. There was little to distinguish them from other goalkeepers. It was likely the function of playing on a good team as compared to a poor team.
When I attempted to write this originally, I noticed that there was a plethora of attacking fullbacks that should be on the National Team.
So, I then looked at the data more closely, and looked at the players in various ways. I subtracted out some items, such as “team dependent” Index 2, and looked at players using Index 1 only, and a few other minor adjustments. But, overall most players remained the same. However, some players shifted 20 points in the rankings, especially for Portland, Washington, and Boston. I could sense that there was something wrong with data.
After a closer inspection of the data, it was time for a sledgehammer…
More from MLS Multiplex
- Javier Milei Elected in Argentina: Potential Impacts on MLS and Signings of Argentine Players
- Orlando City and New York City FC in the Battle for Matías Arezo; Grêmio Enters Negotiations! Who Will Come Out on Top?
- USA, Honduras, Panama, and Canada Close in on a Spot in the 2024 Copa America
- De Gea Turns Down Al-Nassr’s Lucrative Offer: Speculation Points to Possible Reunion with Messi at Inter Miami
- Messi’s Magnetic Impact in the United States
I reread the McHale, Scarf, and Folker paper a couple of times, and found that I made a few errors. I found that the sum of all players scores for Index 1 and Index 2 should equal the same as all of the points in the league. So, when I did this, there was 141 points. Portland having won a bunch of games and had 26 points. Washington had about 18 points, and so on. They were added together to get the 141 points. But originally, the values I had for Indices #1 and #2 were much different, about 200 points for these numbers. So, I made some adjustments and tried again. I found that the Constant of 6.463 was a problem with the Index 1 calculation. Therefore, I am not sure why McHale et al. needed it in the first place.
McHale et al.’s system had some other serious flaws. Using, the Points Sharing system, the “team” i.e. Portland or Boston especially would influence a player’s score by more than 30 to 50 percent of a player’s score in most cases. So, Portland had many players in the top 20, whereas Boston “hung out” in the 80’s or worse. For comparing players across various teams, this is unacceptable and illogical. Goodbye Index number 2.
Also, the Yellow Cards and Red cards had little effect on most teams, but when I removed them, Index 1 looked somewhat better, getting the Index 1 score closer to 141 points.. And the Red Cards inflated the values of certain teams like Portland, Orlando, and Washington. Let us turn those into “zeroes”.
But another problem with the McHale et al calculation is that Crosses were the most important. McHale et al. explained rationally that the theory of the whole index is that certain positive events will lead to shots, shots lead to goals, and goals become victories. This is fine, but with this system, generally attacking fullbacks and wing midfielders are rewarded with crosses. So, I added through balls, launched balls, key passes, and successful corner kicks. All of these passes help the team attack and to me is more logical than just “crosses”.
With those additional passes being added, I had to eliminate the “regular passes” as now that inflated certain players, and “unbalanced” the numbers I had to match above (like the “141 points rule”). Not only that, it was only a 0.034 weighing factor, so it was hardly statistically significant.
I also eliminated the factor of 1.039 for Goals and Assists, but neither affected the overall calculation by a couple of points.
So,after I revised the calculations I came up with this variation- changing “crosses” into “attacking passes”.
Index 1 = (“Attacking Passes” X 0.519) + (Dribbles X 0.118) + (Opp. Interceptions X (-0.024)) + (Opp. Yellow Cards X 0) + (Opp Red Cards X 0) + (Opp Tackle Win Ratio X (-0.170) + (Opp Cleared X (-0.017))+ ( Passes X 0) + Constant of 0.
Note that “Attacking passes” is the sum of Crosses, Through Balls, Key Passes, Launched Balls, and Corner Kicks (all completions, not attempts)
Final Index= 100 X ((Index1 X 0.25) + (Index2 X 0) + (Index3 X 0.125) + (Index4 X 0.125) + (Index5 X 0.0625) + (Index6 X 0.0625).
I wanted to show my new calculation compared to the old calculation to show what I did. This was an effort to maintain as much of the McHale et al. work in place as they had performed the regression statistics on thousands of data points. There was no way, I could replicate anything similar, So, I wanted to keep the “sound statistics” in place while removing those that did not fit in this application.
Let there be another drum roll….
National team players below should have been invited to the Olympics. Definitely!!
- Goalkeepers Hope Solo, Ashlyn Harris, and Alyssa Naeher. For the original McHale et al calculation, and my variations showed nothing that resembled common sense. My system inflated goalies with the high launched ball rate. Therefore for goalkeepers, the comparison is much different. For them, I did a simple Goals Against Average, Save Percentage, and other statistics for the first 10 games of the season. I assume that this was the best way to assess the goalkeepers, as the McHale et al. ranking system did not have any statistics to help goalkeepers other than clean sheets. All 3 goalkeepers had a Goals Against Average of less than 0.70 and save percentage of greater than 82%. The best NWSL goalkeeper overall was Abby Smith of Boston, who unfortunately had a season ending injury early in the season, but her goal against average was 0.50, with over an 87% save percentage. Nicole Barnhart was almost rated about equally with them as well.
- Tobin Heath-She is ranked 2 and her performance on the field at Rio was superior as well. In all calculation variations that I did, Heath was top 6 at all times. This player definitely should have been at the Olympics. But why would Coach Ellis make her play defense at a critical time?
- Crystal Dunn– No goals? No problem! She did not score many goals this year, but fortunately this calculation accounts for other factors. Even with being on a good team like Washington , when you remove the team dependent stats, her worst rank was 16. And yes, she proved it on the Olympic field as well, that she is among the best forwards in the world.
- Mallory Pugh– She gets a free pass as she graduated high school before the Olympics. Her play on the field was among the best. She was one of Coach Ellis’ best decisions for the Olympics. But I cannot statistically prove it.
- Sam Mewis- Ranked 17. With her being an alternate, we did not see her play. But she should have been playing based on these statistics. She had 3 goals and 2 assists the first half of the season, and was among the best at sneaking in Through Balls.
Maybe?
- Kelly O’Hara- When you remove the “team dependent scores”, she jumps up to being ranked number 7. A closer look at her statistics showed that she was heavily involved with the Sky Blue attack completing over 400 regular passes, plus scoring 1 goal and 1 assist. She rates highly on my calculation as she did well in all of the “attacking pass categories”. She obviously gets “bonus points” on the U.S. National because of her versatility. I apologize as in a previous article that I hinted that she did not deserve to be on the National Team, but her statistics are admittedly are better than I thought. But she did not play her best game at the Quarterfinal either. However, the calculation does not look at overall defensive skills either, where on Sky Blue she would be ranked fifth or sixth if you were to only look at defense.
- Ali Krieger– She “picked a bad time” to be stuck on Coach Ellis’ bench. Her overall rank was 38. when you remove the Washington team dependent scores. In all honesty, if I had the skills and time, I would still like to develop a comprehensive rating that equates offense, defense, and overall passing skills to determine the perfect player. My suspicion would be that players like Krieger could be in the top 15. But at this point, I cannot prove it. But it would be a futile exercise, does Coach Ellis even know what defense is nowadays?
- Lindsey Horan– She is ranked 32, and fits in the attacking midfielder or defensive midfielder role. She like Klingenberg and Long fell precipitously when I removed the team dependent stats.
- Allie Long- she surprised me when I looked at statistics with my Portland team review. But when I did the initial calculation she was top 5. However, when I remove the “Portland effects”, she fell to 29.
- Julie Johnston Johnston went up 30 points in the rankings when II added the other passing values. Her good Launched Ball success rate of 53% is compared to 37% for league average.
- Whitney Engen– Engen moved up 50 places on my rating by subtracting out the “Boston points effect” and adding “attacking passes” Only having 4 points for a win and draw destroyed all of the Boston players’ values in the original calculation. Also, like Johnston she is fairly good in the Launched Ball category.
- Alex Morgan– A lot of fans will disagree with this one, but she has a less than desirable ranking of 76. She did score sending it to overtime at the Olympics. But then she missed the penalty kick. The ranking might pick up on the fact that she “disappears” for much of the game, and only scores at opportune times.
- Christen Press– Her numbers looked better than Alex Morgan’s. But she fills the same niche in a way as Morgan and Lloyd. None of the three seem to be involved in much of the attack, but linger around goal and poach in goals from crosses or rebounds. Their scores are lower because other players are doing the “work” for them. McHale et al. admitted that forwards are not properly rewarded in their calculation. Their numbers improved when I added things like Through Balls. But honestly, there not many statistics other than scoring goals and assists to help a forward.
Maybe They Should Have Stayed Home?
- Becky Sauerbrunn – Statistically, when I looked at their calculations,no matter how I performed them Sauerbrunn was at this level (ranked 58 or lower). According to my eyes while watching her play she had a sub par early season, and the statistics show that. She is still a decent center back, but earlier this year, she appeared to be overrated, and that others may have passed her.
- Heather O’Reilly– She was ranked 44.. Like Johnston, she increased in rank when I added passing categories like corner kicks and through balls. But O’Reilly was ranked in the 80’s in all of my previous calculations, except when I added “attacking passes”.
- Megan Klingenberg- She ranked is 36. She was ranked 10 with the original statistics that heavily favored Portland. But her crossing completion rate was below league average. Her lack of pace does not allow for any mistakes. And from what I can tell of her defensive stats, which do not figure much into these calculations, she is average at best. There are other fullbacks ranked ahead of her.
- Emily Sonnett- Sonnett’s overall original score looked good and had her ranked 14. But when remove the team elements of her score and added “attacking passes”, then she falls to 61. Therefore, she was team dependent on her high ranking.
- Morgan Brian– Her low rating is probably due to the hamstring issues that she had in the summer.
These players should have not been invited to the Rio Olympics:
- Carli Lloyd– Sorry Carli, but you should have not been invited to the party! The problem with Lloyd’s low ranking is that she only played 1 full game before being injured in April . However, instead of being out 3 weeks, she did not play until the “friendlies” in July. Lloyd may have been physically fit, but her “team fitness” was sub par as evidenced by poor shot selection and lack of passing skills near the penalty box. In my opinion, the 3 or 4 games she could have played in June should have helped in those areas, in theory. But after her return from the Olympics her passing became much better for Houston in September. But good passing skills are not something you acquire overnight. So, at the Olympics she could have passed to other teammates, but apparently chose not to???
- Megan Rapinoe– No question, this was the worst decision on Coach Jill Ellis’ part. If Rapinoe had to be at the Olympics, it could have been as an alternate. But, there are nearly 200 other people that Ellis could have picked over her. Her physical fitness was horrible to be able to play only 30 minutes..
Maybe Coach Ellis should have invited these other NWSL players to the Olympics instead?
Definitely Should Have Been Considered!
- Vanessa DiBernardo.At 663 points and a number 10 ranking. She is among the best midfielders in the NWSL this year.
- Sarah Killion. She is at 702 points and was ranked 6, and is one of the most underrated players in the league. She and DiBernardo showed up in the top 20 in almost all of my calculations. Both players are quite good at completing Through Balls. Something to maybe break down a Swedish Bunker Defense- eh?
- Lynn Williams. Her points are tepid at 478, putting her about 26th place overall. But, looking at the other forwards about., only Crystal Dunn is ranked higher. Therefore, Williams could have replaced Rapinoe or Lloyd on the roster. But to give you an idea of how comprehensive the overall calculation is, Williams had scored 5 goals and 1 assist with 3 clean sheets for her score. Several other players ranked higher on this list did not score a goal or register an assist. Therefore, in my opinion this is reasonable robust statistical calculation. If the calculation was goal dependent, then Williams would be at the top of the list.
- Lauren Barnes- Defender of the Year 2016. And statistically, that is proven here as well. She had 768 points for an overall rank of 3.
- Abby Dahlkemper – 678 points ranked 8. She gets fairly high rating thanks to Launched Balls and Corner Kicks. Her possession ratio was quite good, she has the skills to dispossess an opponent, and not lose the ball herself.
Definitely – Maybe?
- Casey Short/Arin Gilliland/Elizabeth Eddy.The original rating system strongly favors players who make a lot of crosses per game as it leads to more goals. All of these fullbacks were highly ranked with similar numbers. After other passing statistics were added they fell somewhat. Both Short and Gilliland are top 24. Liz Eddy is a surprise on this list as she was ranked 13. But considering the poor play from the fullbacks at the Olympics, except maybe Krieger who had too much “inexplicable bench time”, maybe 2 or all 3 of them could have made a difference?
- Taylor Lytle- She had a goal and assist early in the season, which brought her rating up to a 542, which was 21 overall. Unfortunately for her, later in the season , she was overshadowed by players like Leah Galton and Sam Kerr. Most of Lytle’s numbers reflect that she filled her role adequately before they arrived on the team.
And this player was born in the wrong country…
Kim Little! The Scottish National Team heroine was ranked 1 overall, with a score 922. She is our number 1 choice for the U.S. National Team! And she was ranked top 4 in all calculations.
And in conclusion, the system that McHale, Scarf. and Folker proposed may be fine for their application at EA Sports®. However, I found that with the main index heavily dependent of Crosses, it favored the attacking fullback and wing midfielder, while ignoring other midfielders and forwards.I tried to modify the calculation to be more inclusive of other players with some mixed success. However,I consider that this particular index is not useful for goalkeepers in general, and that maybe a seventh index be added for them, somehow incorporating save percentage and goals against average.
I hope the U.S. Soccer Federation has a decent set of metrics to evaluate players for the 2019 World Cup and 2020 Olympics. I think fans around the country would like to think that the best players were chosen to be there, instead on counting on those players with old past glories…
Sources:
- McHale, Scarf, and Folker: Soccer Player Performance Rating System , 2012 “On the Development of a Soccer Player Performance Rating System for the English Premier League” Interfaces Vol. 42, No. 4, July–August 2012, pp. 339–351 ISSN 0092-2102 (print)—ISSN 1526-551X (online). ©2012 INFORMS
- I have acquired about a half season’s worth of 2016 NWSL data from Alfredo Martinez, who has https://wosostats.wordpress.com and I also took the data from his report here.
- Some data is also taken from the NWSL site